操作场景
本文将演示如何使用 GPU 云服务器优化 AI 绘画模型,模型范围包括以 Stable Diffusion 1.5为基础的系列模型,您可以使用 Lora 结合模型使用,支持ControlNet。TACO Infer 的加速能力优化后,端到端时延可减少约30%~50%。
操作步骤
购买 GPU 云服务器
实例:选择 计算型 PNV4。
系统盘:配置容量不小于500GB的云硬盘。
镜像:建议选择公共镜像。
操作系统使用 CentOS 7.9。
选择公共镜像后请勾选后台自动安装 GPU 驱动,实例将在系统启动后预装对应版本驱动。如下图所示:
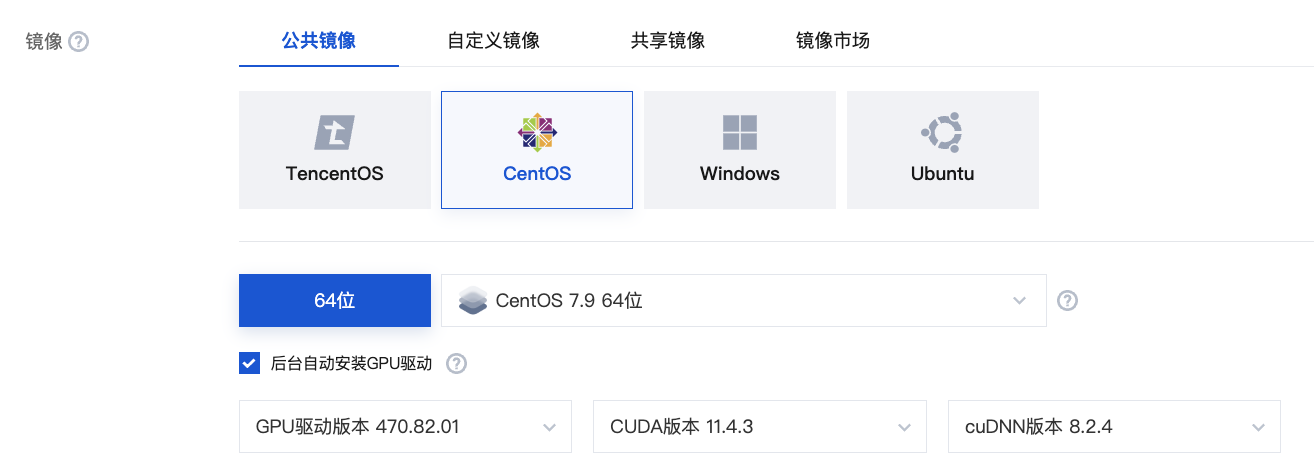
注意:
当前优化版本仅支持 A10、A100、V100 GPU 机型,请检查您的实例配置。
优化过程会有中间状态文件产生,建议您系统盘容量不小于500GB。
安装 docker 和 NVIDIA docker
1. 参见 使用标准登录方式登录 Linux 实例,登录实例。
2. 执行以下命令,安装 docker。
curl -s -L http://mirrors.tencent.com/install/GPU/taco/get-docker.sh | sudo bash
3. 执行以下命令,安装 nvidia-docker2。
curl -s -L http://mirrors.tencent.com/install/GPU/taco/get-nvidia-docker2.sh | sudo bash
下载并启动 docker 镜像
docker pull xxxxxxxxdocker run -it --rm --gpus=all --network=host xxxxxxxx
说明:
docker 镜像近作为优化模型步骤演示,部署在生产环境中需要您自制镜像,TACO Infer 提供软件安装包。
环境准备
1. 进入
/root/sd_webui_demo/stable-diffusion-webui
目录中,执行:bash webui.sh
脚本会自动构建 python virtualenv 环境,安装 stable-diffusion-webui 所有相关依赖,完成后您可以退出进程。
2. 进入
/root/sd_webui_demo
目录中,执行以下令安装 TACO Infer,下载模型权重:source env.sh
说明:
env.sh 文件中提供示例模型下载,如果您需要下载自己模型,需要修改脚本中下载路径。
如果您重新进入容器,可以执行 source /root/sd_webui_demo/stable-diffusion-webui/venv/bin/activate 激活虚拟环境。
(可选)合并LoRA到模型中
1. 进入
/root/sd_webui_demo/stable-diffusion-v1-5
目录中,使用 git clone https://github.com/kohya-ss/sd-scripts
命令,下载合并 LoRA 与基础模型的脚本库。2. 进入
/root/sd_webui_demo/stable-diffusion-v1-5/sd-scripts
目录,配置环境变量:export PYTHONPATH=/root/sd_webui_demo/stable-diffusion-v1-5/sd-scripts
3. 参见以下命令合并 LoRA 与基础模型:
python networks/merge_lora.py --sd_model ../v1-5-pruned-emaonly.safetensors --save_to ../lora-v1-5-pruned-emaonly.safetensors --models <LoRA文件目录> --ratios 0.8
模型导出
1. 执行 python export_model.py,用 diffusers 加载模型权重,并导出为 torchscript,原文件内容如下,注意修改相应的部分:
import torchimport functoolsfrom diffusers import StableDiffusionPipelinedef get_unet(device="cuda:0"):# change to your model pathmodel_path = "./stable-diffusion-v1-5"# change if your model merged with LoRA# model_path = "./stable-diffusion-v1-5/lora-v1-5-pruned-emaonly.safetensors"# pipe = StableDiffusionPipeline.from_ckpt(model_path).to(device)pipe = StableDiffusionPipeline.from_pretrained(model_path).to(device)unet = pipe.unetunet.eval()unet.to(memory_format=torch.channels_last) # use channels_last memory formatunet.forward = functools.partial(unet.forward, return_dict=False) # set return_dict=False as defaultreturn unetdef get_sample_input(batch_size, latent_height, latent_width, device="cuda:0"):dtype = torch.float32text_maxlen = 77embedding_dim = 768return (torch.randn(2*batch_size, 4, latent_height, latent_width, dtype=dtype, device=device),torch.tensor([1.]*batch_size, dtype=dtype, device=device),torch.randn(2*batch_size, text_maxlen, embedding_dim, dtype=dtype, device=device))model = get_unet()# change according to your image (1, h/8, w/8)test_data = get_sample_input(1, 64, 64)script_model = torch.jit.trace(model, test_data, strict=False)script_model.save("origin_model/trace_module.pt")
注意:
如果是单文件格式(ckpt 或 safetensors)或您使用合并 LoRA 后的模型,请修改 pipe = StableDiffusionPipeline.from_pretrained(model_path).to(device) 为 pipe = StableDiffusionPipeline.from_ckpt(model_path).to(device)。
2. 进入
origin_model
目录下可以查看导出的模型:[root@vm-0-46-centos demo]# ll origin_model/total 3358720-rw-r--r-- 1 500 500 3439324538 Mar 13 16:56 trace_module.pt
模型优化
1. 执行
python demo.py
命令即可启动 TACO Infer 对导出的 UNetModel 进行性能优化,注意参见注释修改 demo.py 文件:import torchfrom taco import optimize_gpu, ModelConfig, OptimizeConfigdef gen_test_data(batch_size=1):# change according to your image (1, h/8, w/8)x = torch.randn(batch_size, 4, 64, 64)timesteps = torch.tensor([1]*batch_size)# change according to your model hidden sizecontext = torch.randn(batch_size, 77, 768)return (x, timesteps, context)input_model = "origin_model/trace_module.pt"output_model_dir = "./optimized_model"optimize_config = OptimizeConfig()optimize_config.pytorch.trt.enable_fp16 = Trueoptimize_config.pytorch.profiling.subproc = Falseoptimize_config.profiling.num_run = 50optimize_config.profiling.num_warmup = 10model_config = ModelConfig()model_config.pytorch.large_model=True# change according to your image (h/8, w/8)# change according to your model hidden sizemodel_config.pytorch.inputs_shape_range = [{"min": (1, 4, 64, 64), "opt": (2, 4, 64, 64), "max": (32, 4, 64, 64)},{"min": (1,), "opt": (2,), "max": (32,)},{"min": (1, 77, 768), "opt": (2, 77, 768), "max": (32, 77, 768)},]report = optimize_gpu(input_model,output_model_dir,test_data=gen_test_data(batch_size=2),optimize_config = optimize_config,model_config = model_config)
docker 镜像内默认优化代码是 A10 的优化代码,如果您在 V100 机型上使用,请使用以下代码进行优化:
import osimport torchfrom taco import optimize_gpu, ModelConfig, OptimizeConfigdef gen_test_data(batch_size=1):dtype = torch.float16device = "cuda:0"# change according to your image (1, h/8, w/8)x = torch.randn(batch_size, 4, 64, 64, dtype=dtype)timesteps = torch.tensor([1]*batch_size, dtype=dtype)# change according to your model hidden sizecontext = torch.randn(batch_size, 77, 768, dtype=dtype)return (x,timesteps,context,torch.randn(batch_size, 1280, 8, 8, dtype=dtype), # control net mid_residualtorch.randn(batch_size, 320, 64, 64, dtype=dtype), # control net down_residual0torch.randn(batch_size, 320, 64, 64, dtype=dtype), # control net down_residual1torch.randn(batch_size, 320, 64, 64, dtype=dtype), # control net down_residual2torch.randn(batch_size, 320, 32, 32, dtype=dtype), # control net down_residual3torch.randn(batch_size, 640, 32, 32, dtype=dtype), # control net down_residual4torch.randn(batch_size, 640, 32, 32, dtype=dtype), # control net down_residual5torch.randn(batch_size, 640, 16, 16, dtype=dtype), # control net down_residual6torch.randn(batch_size, 1280, 16, 16, dtype=dtype), # control net down_residual7torch.randn(batch_size, 1280, 16, 16, dtype=dtype), # control net down_residual8torch.randn(batch_size, 1280, 8, 8, dtype=dtype), # control net down_residual9torch.randn(batch_size, 1280, 8, 8, dtype=dtype), # control net down_residual10torch.randn(batch_size, 1280, 8, 8, dtype=dtype), # control net down_residual10)input_model = "origin_model/trace_module.pt"output_model_dir = "./optimized_model"optimize_config = OptimizeConfig()optimize_config.pytorch.tidy.enable = Falseoptimize_config.pytorch.trt.enable_fp16 = Trueoptimize_config.pytorch.profiling.subproc = Falseoptimize_config.profiling.num_run = 50optimize_config.profiling.num_warmup = 10os.environ["TORCHTRT_DISABLE_FMHA"] = "1"os.environ["TORCHTRT_DISABLE_FMHCA"] = "1"model_config = ModelConfig()model_config.pytorch.large_model=True# change according to your image (h/8, w/8)# change according to your model hidden sizemodel_config.pytorch.inputs_shape_range = [{"min": (1, 4, 64, 64), "opt": (2, 4, 64, 64), "max": (8, 4, 64, 64)},{"min": (1,), "opt": (2,), "max": (8,)},{"min": (1, 77, 768), "opt": (2, 77, 768), "max": (8, 77, 768)},{"min": (1, 1280, 8, 8), "opt": (2, 1280, 8, 8), "max": (8, 1280, 8, 8)},{"min": (1, 320, 64, 64), "opt": (2, 320, 64, 64), "max": (8, 320, 64, 64)},{"min": (1, 320, 64, 64), "opt": (2, 320, 64, 64), "max": (8, 320, 64, 64)},{"min": (1, 320, 64, 64), "opt": (2, 320, 64, 64), "max": (8, 320, 64, 64)},{"min": (1, 320, 32, 32), "opt": (2, 320, 32, 32), "max": (8, 320, 32, 32)},{"min": (1, 640, 32, 32), "opt": (2, 640, 32, 32), "max": (8, 640, 32, 32)},{"min": (1, 640, 32, 32), "opt": (2, 640, 32, 32), "max": (8, 640, 32, 32)},{"min": (1, 640, 16, 16), "opt": (2, 640, 16, 16), "max": (8, 640, 16, 16)},{"min": (1, 1280, 16, 16), "opt": (2, 1280, 16, 16), "max": (8, 1280, 16, 16)},{"min": (1, 1280, 16, 16), "opt": (2, 1280, 16, 16), "max": (8, 1280, 16, 16)},{"min": (1, 1280, 8, 8), "opt": (2, 1280, 8, 8), "max": (8, 1280, 8, 8)},{"min": (1, 1280, 8, 8), "opt": (2, 1280, 8, 8), "max": (8, 1280, 8, 8)},{"min": (1, 1280, 8, 8), "opt": (2, 1280, 8, 8), "max": (8, 1280, 8, 8)},]report = optimize_gpu(input_model,output_model_dir,test_data=gen_test_data(batch_size=2),optimize_config = optimize_config,model_config = model_config)
说明:
优化过程中“ [INFO] ERROR: [Torch-TensorRT TorchScript Conversion Context] - 2: [virtualMemoryBuffer.cpp::resizePhysical::145] Error Code 2: OutOfMemory (no further information)”提示可忽略。
2. 优化完成后,会输出性能优化报告,格式如下:
{"hardware": {"device": "NVIDIA A10, driver: 470.82.01","driver": "470.82.01","num_gpus": "1","cpu": "AMD EPYC 7K83 64-Core Processor, family '25', model '1'"},"software": {"taco version": "0.2.33","framework": "pytorch","framework version": "1.12.1+cu113","torch device": "NVIDIA A10"},"summary": {"working directory": "/root/sd_webui_demo","input model path": "origin_model/trace_module.pt","output model folder": "./optimized_model","input model format": "torch.jit saved (traced or scripted) model","status": "satisfactory","baseline latency": "191ms 393us","accelerated latency": "40ms 707us","speedup": "4.70","optimization time": "19min 39s 416ms","env": "{}"}}
在 optimized_model 目录中查看优化后的模型:
[root@4e302835766c /root/demo]#ll optimized_model/total 1.8Gdrwxr-xr-x 2 500 500 4.0K Mar 3 14:38 ./-rw-r--r-- 1 500 500 1.8G Mar 13 16:46 optimized_recursive_script_module.ptdrwxr-xr-x 7 500 500 4.0K Mar 13 16:47 ../
(可选)模型验证
经过以上步骤,得到优化后的模型文件之后,您可以使用 torch.jit.load 接口加载该模型,验证其性能和正确性。加载模型运行的示例代码如下所示:
import torchimport tacoimport ostaco_path = os.path.dirname(taco.__file__)torch.ops.load_library(os.path.join(taco_path, "torch_tensorrt/lib/libtorchtrt.so"))optimized_model = torch.jit.load("optimized_recursive_script_module.pt")pic = torch.rand(1, 4, 64, 64).cuda() // picturetimesteps = torch.tensor([1]*1) // timestepscontext = torch.randn(1, 77, 768) // text embeddingwith torch.no_grad():output = optimized_model(pic, timesteps, context)print(output)
需要注意的是,由于优化后的模型包含经过高度优化的 TACO Kit 自定义算子,因此运行模型之前,需要执行
import taco
加载包含自定义算子的动态链接库。您根据自己的输出模型目录调整好相关参数之后,运行以上代码,即可加载优化后的模型进行推理计算。
启动优化后的模型
修改 webui-user.sh 脚本后,使用 bash webui.sh 命令启动 webUI 服务,将会自动加载 TACO Infer 优化后模型:
# edit webui-user.sh# Uncomment to enable TACO opt UNetModelexport TACO_OPT_UNET="/root/sd_webui_demo/optimized_model/optimized_recursive_script_module.pt"bash webui.sh
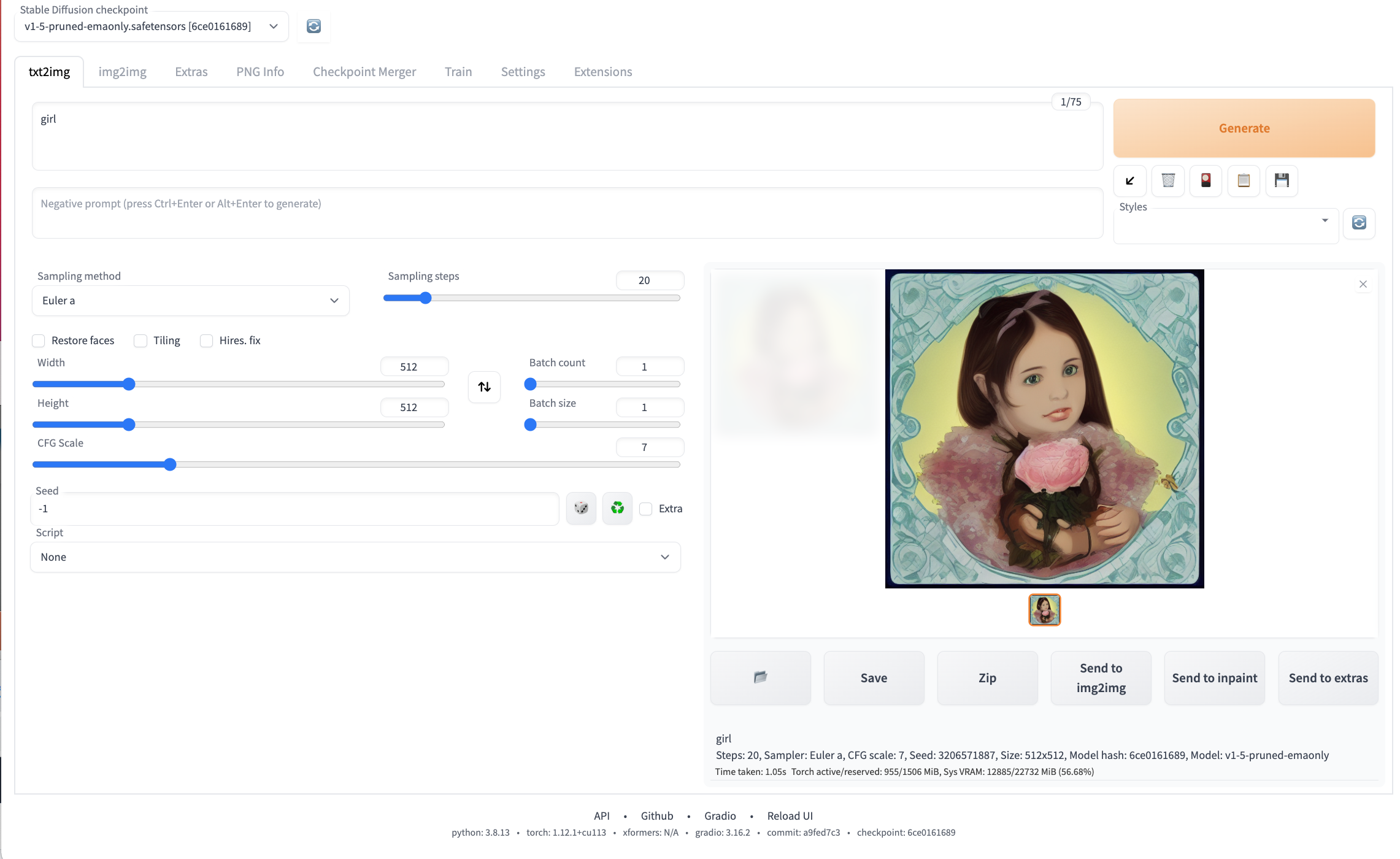
可以看到使用 TACO Infer 优化后,单张 A10 卡生成一张512 × 512图片时间仅为1秒左右。
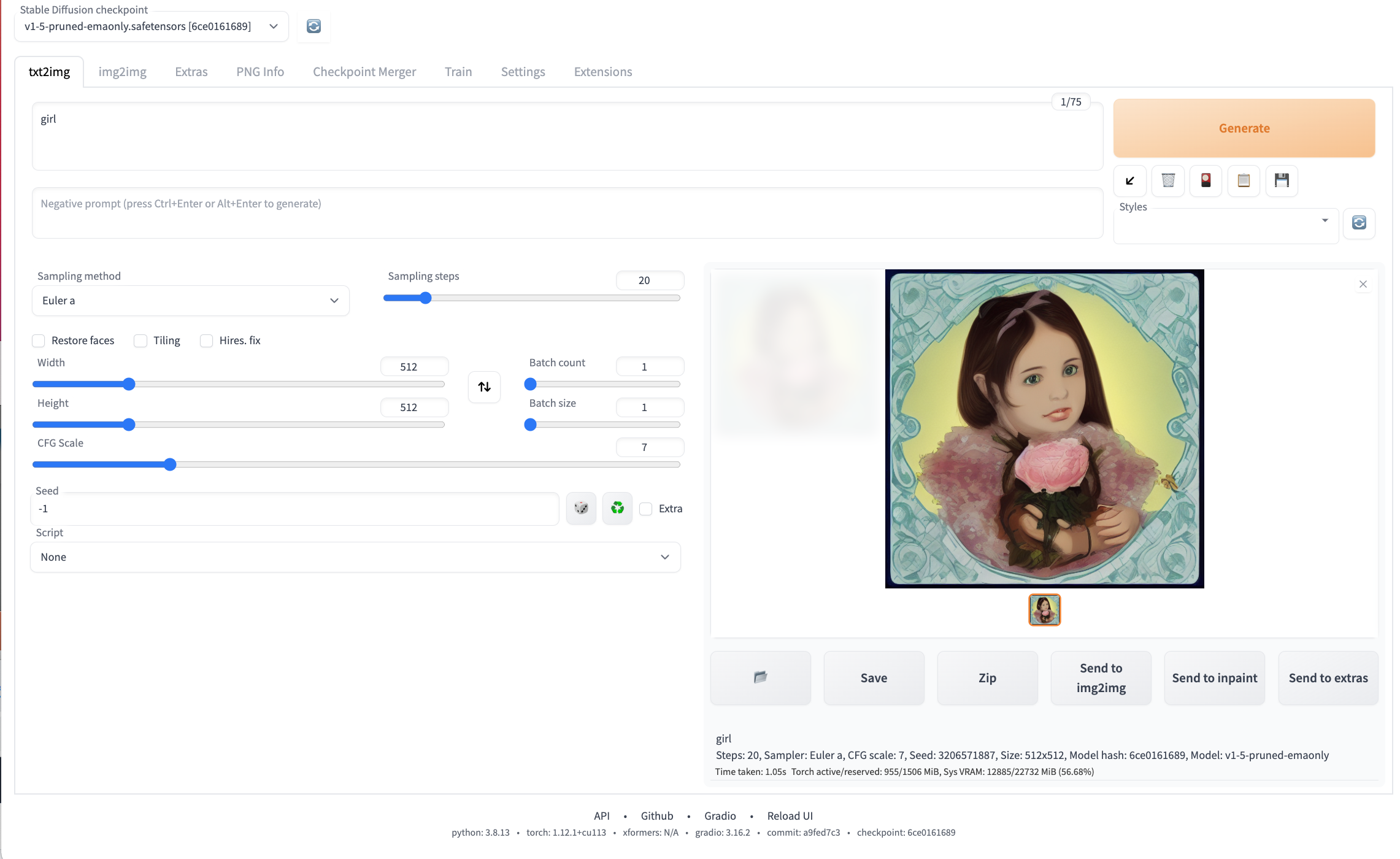
总结
本文基于腾讯云 GPU 云服务器评测优化了 Stable diffusion 模型,通过TACO Infer的优化,在模型耗时主体结构 Unet 上获得了超过 4 倍的性能提升,端到端时延减少一半,助力业务性能大幅提升,吞吐率翻倍。